Exploring the world of AI
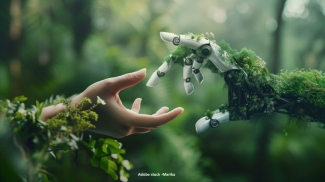
The Young Scientists Session at the EFI Annual Conference took a deep dive into the world of artificial intelligence, exploring not only its challenges but also the opportunities it may generate for forest-related research.
The growing role of AI has been widely discussed in society and the media, with many concerned about transparency issues, data bias and discrimination, malicious use and privacy, security risks as well as environmental costs. However, it’s also increasingly used in forest-related research (for example in mapping or monitoring).
The interactive session began with Gabriele Colombo from Politecnico di Milano, presenting the results of a Hackathon on Forests and generative AI, held on 17 September. Bringing together participants from science, media and civil society, the Hackathon tested how different generative AI text-to-image models interpreted complex forest-related subject matter. By prompting the models (MidJourney, DeepAI, Dall-e 3 and Stable Diffusion) with phrases like “A photo of foresters in the Amazon forest” or “A forested park in Warsaw”, Hackathon participants attempted to look into the ‘black box’ of the AI model and explore perceptions and biases.
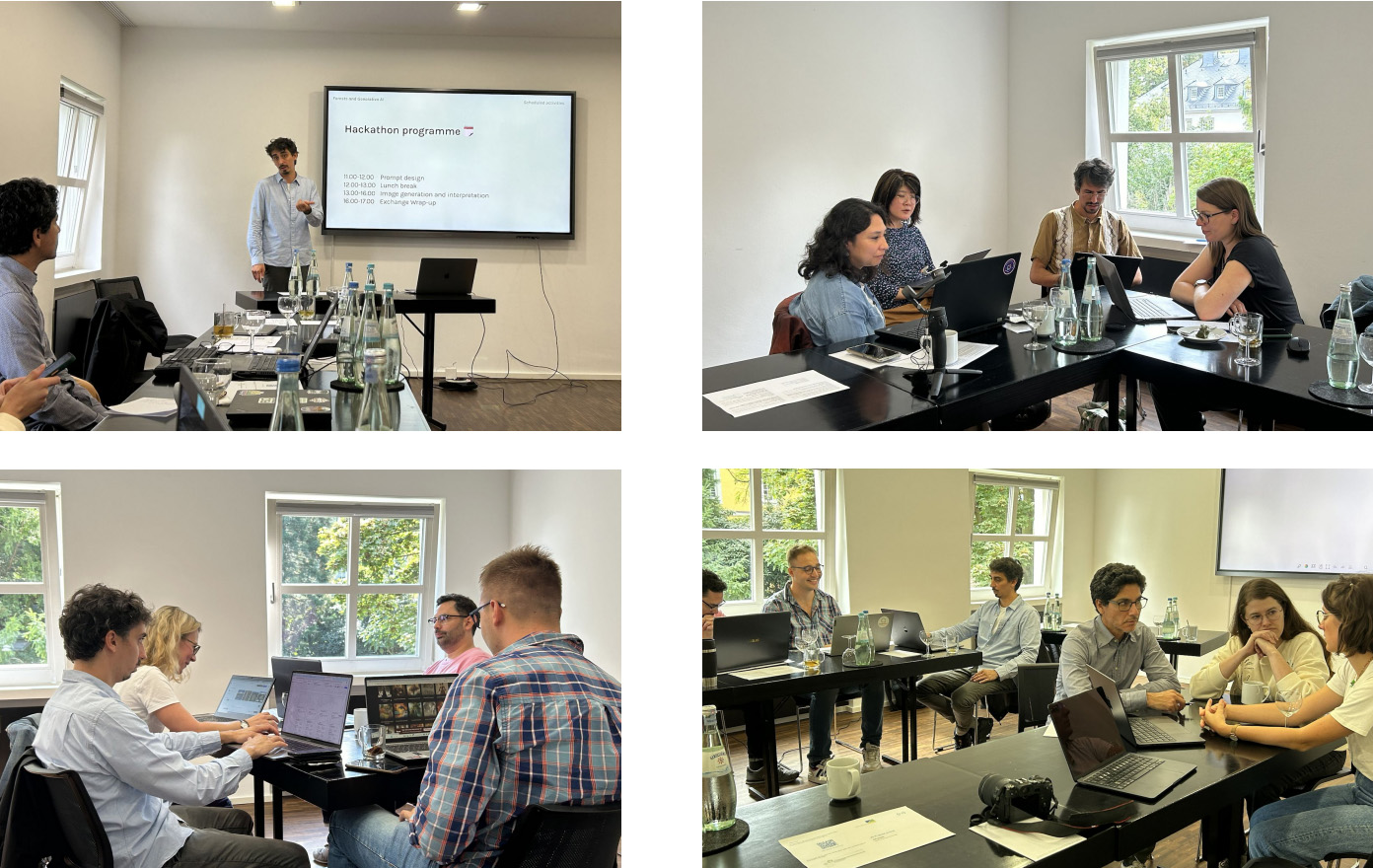
The Annual Conference participants also had a go at interpreting the images created, exploring some of the stereotypes and patterns seen in the images. The session demonstrated how this method of collaborative interpretation has good potential for multistakeholder engagement, learning how different groups of people from different backgrounds perceive different issues with the forest images.
Three Young Scientists received grants from EFI to attend the EFI Annual Conference, and showcase the work they are doing with AI.
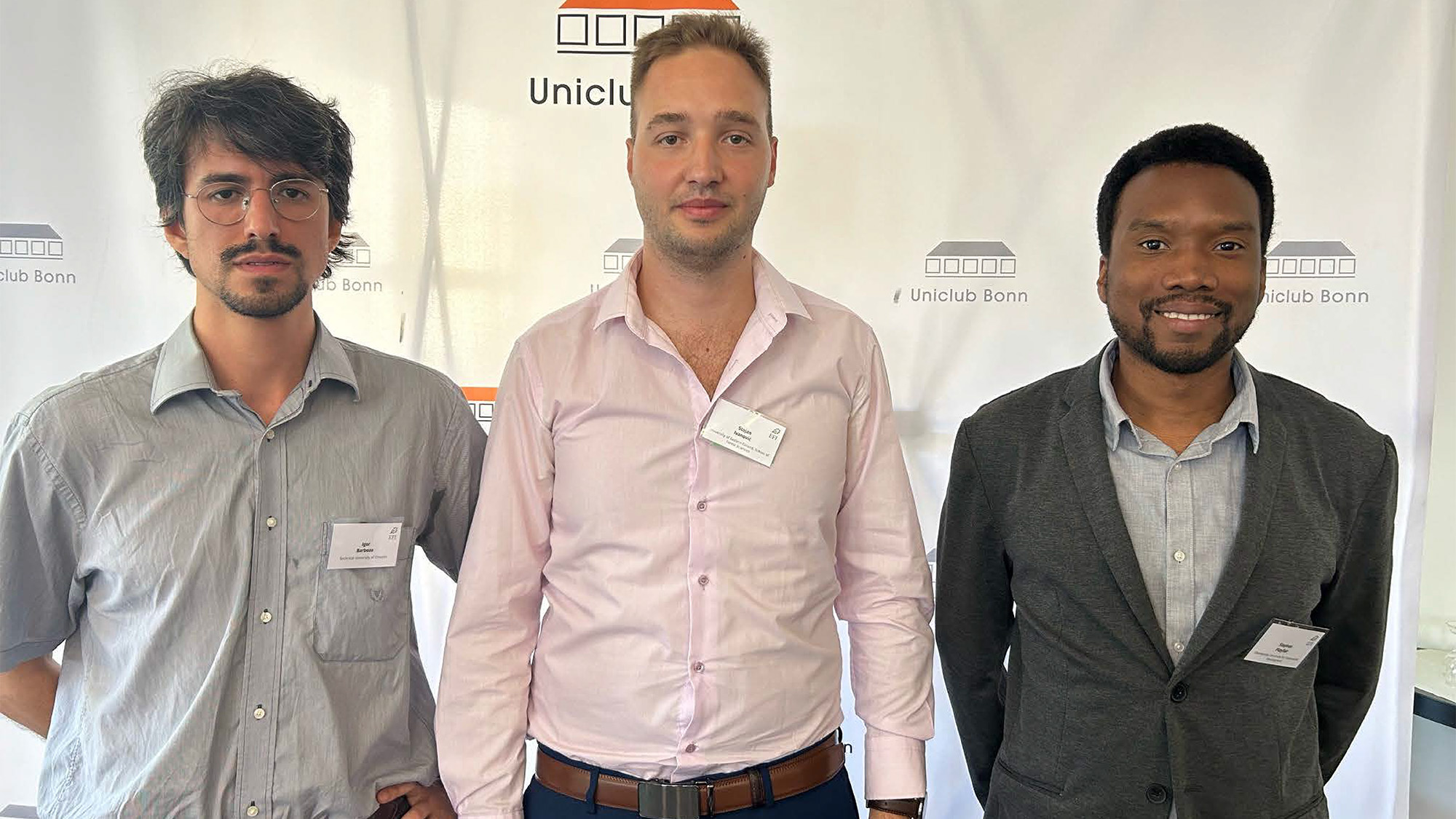
Igor Felipe de Souza Barboza, Stojan Ivanovic, Stephan Playfair
Stephan Playfair from Eberswalde University for Sustainable Development (HNEE) presented the possibilities for an AI-enabled forest system platform. The challenge with interacting with Large Language Models (LLMs) is that they don’t have access to real-world data, and are prone to hallucinate or guess an answer. Stephan showed how his work is training a model with its own knowledge base (c.500 forest research papers) and forest-related data (for example tree location/height), to make the model smarter. The model should be ready in 2025 – potential practical applications could include helping to make decisions on how to manage a forest adapted to climate change.
Stojan Ivanovic from the University of Eastern Finland explored how AI could help with automating mapping and classifying boreal forests. Convolutional Neural Networks are a deep learning model that can process and make predictions from data – in this case identifying broadleaf forests. Using aerial images from across Finland, and taking account the fact that broadleaf trees have a higher reflectance than coniferous trees, the model was used to classify and map the forest. This is a cost-effective and fast methods, which could be useful for large-scale ecological mapping. However, it needs a supercomputing processor, and at present has insufficient precision for forest industry inventories.
Igor Felipe de Souza Barboza from the Technical University of Dresden presented an evaluation of AI and enabling technologies for social development and forest conservation in the Amazon. Interviews with 35 stakeholders and a literature review showed many applications: 39 AI and machine learning technologies, 31 data acquisition technologies, 19 data governance and interface technologies and 14 connecting and infrastructure. Opportunities for use included biodiversity assessments which could be made faster, and more accurate with easier logistics, for example by using drone technology, or bioacoustics to complement data collection. However, challenges include internet connectivity in remote areas, and impacts on society.
Photos: Shanqing Gao, Satu Ikonen-Williams